Data Lakehouse vs. Data Warehouse: Choosing the Right Foundation for Your Data Strategy
In today’s data-driven world, organisations are constantly searching for the best way to manage, store, and analyse their data. Two common approaches that come up in these discussions are data lakehouse vs data warehouse. However, the differences between them can be confusing, leaving business leaders uncertain about which solution to adopt for their unique needs.
In this post, we’ll clarify what makes data warehouses and data lakehouses distinct, explore the benefits and drawbacks of each, and provide guidance to help you make the right choice for your data strategy.
What is a Data Warehouse?
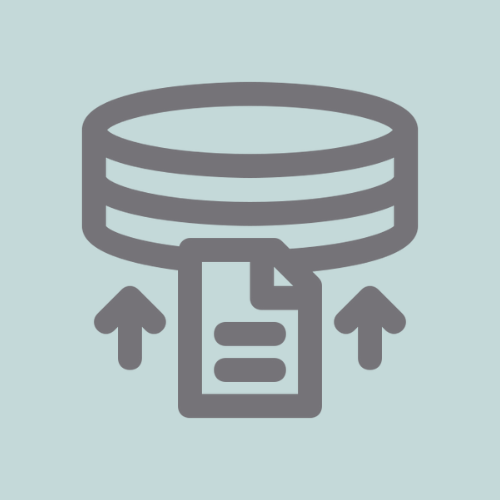
A data warehouse is a centralised repository designed to store large volumes of structured data — highly organised information typically stored in tables with predefined fields, such as names or transaction amounts. It organises and optimises data for business intelligence (BI) and reporting, allowing users to derive insights from historical data in a reliable and efficient way. Cloud services like Azure SQL Database provide scalable and secure solutions for building, managing, and maintaining data warehouses.
Architecture of a Data Warehouse
Data warehouses are built on an Extract, Transform, Load (ETL) process, where data is extracted from source systems, transformed into a standardised format, and then loaded into the warehouse. This structure supports organises data storage and fast query performance.
Pros of a Data Warehouse
- High Performance: Optimised for fast query processing, making it ideal for analytics.
- Structured Data Handling: Organises structured data effectively, ensuring consistency and reliability.
- Business Intelligence (BI) Support: Commonly used for BI and reporting tasks, providing insights into historical data.
Cons of a Data Warehouse
- Costly: Data warehouses can be expensive to build and maintain, especially at large scales.
- Limited Flexibility: They are less effective at handling unstructured data (e.g., text, video).
- Complex ETL Process: Rigid ETL processes can limit the speed and flexibility of data ingestion and management.
What is a Data Lakehouse?
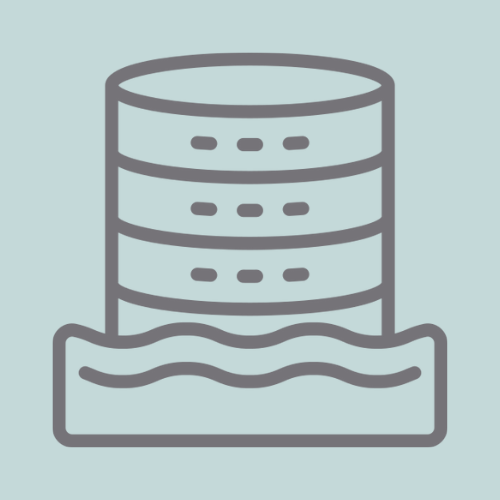
A data lakehouse is a hybrid approach that combines elements of data warehouses and data lakes. Data lakes are large, centralised storage systems that hold vast amounts of raw data in its original format, and can handle both structured and unstructured data, making them a more versatile solution for organisations working with diverse data types. Lakehouses also support advanced analytics and machine learning workloads, which require a mix of data types and flexibility. Cloud services like Databricks and Microsoft Fabric provide the tools to build and manage scalable data lakehouses, offering flexibility for both structured and unstructured data.
Architecture of a Data Lakehouse
Data lakehouses are based on an Extract, Load, Transform (ELT) process, where data is loaded into the lakehouse in its raw form and then transformed as needed. This allows for scalability and cost-effectiveness, especially for large-scale data storage.
Pros of a Data Lakehouse
- Flexible Data Handling: Manages structured, semi-structured, and unstructured data within a single platform.
- Cost-Effective: Reduces storage costs by enabling raw data storage and on-demand transformations.
- Real-Time and Advanced Analytics: Supports a variety of workloads, including real-time analytics and machine learning.
Cons of a Data Lakehouse
- Complex Management: Integration and data governance can be challenging, as it requires more complex processes.
- Newer Technology: The technology is still evolving, and standard practices are still being defined.
Comparing Data Lakehouse and Data Warehouse
Understanding the key differences between data lakehouses and data warehouses can help you choose the best fit for your organization.
Data Structure and Storage
- Data Warehouse: Structured data only, with a rigid schema.
- Data Lakehouse: Supports all data types, from structured to unstructured, with flexible schema options.
Performance and Scalability
- Data Warehouse: Optimised for structured data and complex queries but can become costly to scale.
- Data Lakehouse: Scalable and cost-effective, particularly for large, diverse datasets.
Cost Implications
- Data Warehouse: Higher storage and management costs.
- Data Lakehouse: Lower storage costs by allowing raw data storage and on-demand processing.
Integration and Management
- Data Warehouse: Straightforward integration for BI tasks, but struggles with unstructured data.
- Data Lakehouse: More complex integration, but versatile for a wide array of data types and workloads.
Decision Criteria: Choosing the Right Solution
Here are some guidelines for determining which solution is best for your organization:
- Data Types: If you primarily work with structured data for BI purposes, a data warehouse may be the better choice. For mixed data types (e.g., text, images, etc.), consider a data lakehouse.
- Budget: Data warehouses are known for high operational costs, while data lakehouses offer more economical options, particularly for unstructured or raw data.
- Use Cases: For real-time analytics, machine learning applications, or AI models that require large, varied datasets to deliver advanced insights and predictions, a data lakehouse is often the more suitable choice due to its versatility. Traditional reporting tasks, however, may still benefit from the reliability and structured approach of a data warehouse.
Use Cases
When to Use a Data Warehouse
- High-Performance Analytics on Structured, Stable Data: If your organisation requires efficient querying of structured data with consistent performance, a data warehouse is ideal. Its architecture is designed for rapid processing of complex queries, making it well-suited to handle stable datasets that need routine and highly performant analysis—such as financial or operational reporting across thousands of transactions.
- Business-Critical, Low-Latency Reporting: For industries where reporting needs to happen with minimal delay and utmost reliability (like real-time financial reporting), data warehouses can outperform lakehouses. They are typically better optimised for low-latency responses and predictable performance, especially when handling high volumes of structured data in environments where delays could impact decision-making.
- Simplified Data Governance for Legacy BI Systems: Organisations with mature, legacy BI tools that rely on highly structured, cleaned data often benefit from data warehouses. The ETL processes in a data warehouse support stringent data quality standard, simplifying data governance and integration with legacy systems.
When to Use a Data Lakehouse
- Customer Analytics Across Diverse Data Sources: Businesses today want a 360-degree view of their customers, pulling insights from web data, social media, purchase history, and more. A data lakehouse’s flexibility with unstructured data makes it ideal for these diverse datasets, which can support more holistic, real-time customer analytics.
- Machine Learning and AI: Data lakehouses are well-suited for machine learning and artificial intelligence projects, where the ability to work with both structured and unstructured data is critical. A data lakehouse enables organisations to store raw data and conduct complex analyses without the costly preprocessing typical in data warehouses.
- Scalable, Cost-Efficient Big Data Storage: If your organization needs to store massive volumes of data at a lower cost while still allowing access to real-time insights, a data lakehouse provides a flexible and scalable solution. It enables long-term storage of raw data, ready to be analyzed or transformed as needed.
For more information on data engineering solutions, visit our Data Engineering Services page.
Future Trends
Evolution of Data Lakehouses
Data lakehouses are expected to become more advanced, with improvements in real-time analytics capabilities and deeper integration with machine learning platforms. These advancements will make lakehouses an increasingly attractive option for organisations working with diverse data.
Future Developments in Data Warehouses
Data warehouses are also evolving, with trends leaning toward cloud-native solutions that bring down costs and improve scalability.
Impact on Data Management Strategies
As data needs grow, organisations may adopt a hybrid approach, combining the strengths of both data warehouses and lakehouses. This allows them to leverage structured data for BI while also tapping into the flexibility of unstructured data in lakehouses.
Conclusion
Choosing between a data lakehouse and a data warehouse is a strategic decision that should align with your data management goals, data diversity, and budget. While data warehouses remain invaluable for structured analytics, data lakehouses offer flexibility for a variety of data types and applications.
Ready to explore your options? Connect with our team to discuss how we can help tailor the best data solution for your business needs.
Ready to explore your options?
Connect with our team to discuss how we can help tailor the best data solution for your business needs.